Prometheus 是一种越来越受欢迎(理由充分)的开源工具,它为应用程序和服务器提供监控和告警功能。 Prometheus 的强大之处在于监控服务器端指标,它将其存储为时间序列数据。虽然 Prometheus 不适合应用程序性能管理、主动控制或用户体验监控(尽管 GitHub 扩展确实使用户浏览器指标可用于 Prometheus),但其作为监控系统的强大功能以及通过服务器联合实现高可扩展性的能力使 Prometheus 成为各种用例的强大选择。
在本文中,我们将更仔细地了解 Prometheus 的架构和功能,然后检查该工具在运行中的详细实例。
Prometheus 架构和组件
Prometheus 包括 Prometheus 服务器(处理服务发现、指标检索和存储,以及通过 PromQL 查询语言进行的时间序列数据分析)、指标的数据模型、图形 GUI 以及对 Grafana 的原生支持。 还有一个可选的告警管理器,允许用户通过查询语言定义告警,以及一个可选的推送网关,用于短期应用程序监控。 这些组件的位置如下图所示。
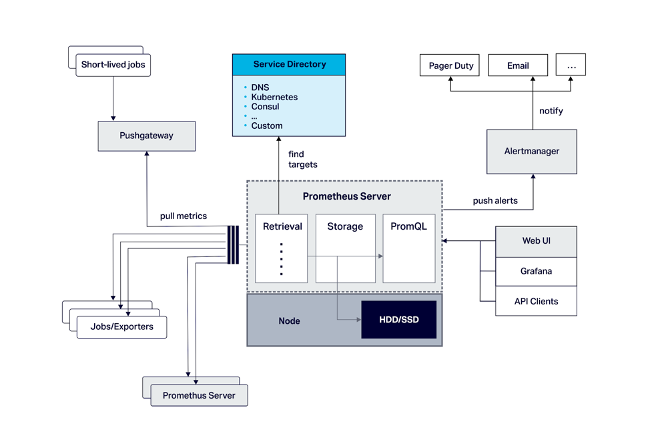
Prometheus 可以通过使用代理在应用程序环境中执行通用代码来自动捕获标准指标。 它还可以通过检测捕获自定义指标,将自定义代码放置在受监控应用程序的源代码中。 Prometheus 官方支持 Go、Python、Ruby 和 Java/Scala 的 客户端库,并且还允许用户编写自己的库。 此外,还有许多其他语言的非官方库可用。
开发人员还可以利用第三方 exporter 来自动激活他们可能正在使用的许多流行软件解决方案的检测。 例如,使用基于 JVM 的应用程序(如开源 Apache Kafka 和 Apache Cassandra)的用户可以通过利用现有的 JMX exporter 轻松收集指标。 在其他情况下,可能不需要 exporter,因为应用程序将公开已经是 Prometheus 格式的指标。 Cassandra 的用户可能还会发现 Instaclustr 免费提供的 Cassandra Exporter for Prometheus 很有用,因为它将来自自我管理的集群的 Cassandra 指标集成到 Prometheus 应用程序监控中。
同样重要的是:开发人员可以利用可用的 node exporter 来监控内核指标和主机硬件。 Prometheus 还提供了一个 Java 客户端,它具有许多功能,可以零散地注册,也可以通过单个 DefaultExports.initialize(); 命令一次性注册——包括内存池、垃圾收集、JMX、类加载和线程计数。
Prometheus 数据建模和指标
Prometheus 提供四种指标类型
- Counter(计数器):计算递增的值; 重新启动可以将这些值重置为零
- Gauge(仪表):跟踪可以上升和下降的指标
- Histogram(直方图):根据指定的响应大小或持续时间观察数据,并计算观察到的值的总和以及可配置存储桶中的计数
- Summary(摘要):计算与直方图类似观察到的数据,并提供在滑动时间窗口内计算的可配置分位数
Prometheus 时间序列数据指标每个都包含一个字符串名称,该名称遵循命名约定,以包括受监控数据主题的名称、逻辑类型和使用的度量单位。 每个指标都包含 64 位浮点值的流,这些值的时间戳精确到毫秒,以及一组标记其测量维度的键:值对。 Prometheus 自动将 Job 和 Instance 标签添加到每个指标,以跟踪数据目标的配置作业名称和抓取目标 URL 的 <host>:<port> 部分。
Prometheus 示例:Anomalia Machina 异常检测实验
在进入示例之前,请按照此入门指南下载并开始使用开源 Prometheus。
为了演示如何将 Prometheus 投入使用并以高规模执行应用程序监控,让我们看一下我们在 Instaclustr 完成的最近的 实验性 Anomalia Machina 项目。 该项目(只是一个测试用例,而不是商业上可用的解决方案)利用 Kubernetes 部署的应用程序中的 Kafka 和 Cassandra,该应用程序对流数据执行异常检测。 (这种检测对于包括物联网应用程序和数字广告欺诈在内的用例至关重要。)实验性应用程序严重依赖 Prometheus 来收集跨分布式实例的应用程序指标,并使其易于查看。
下图显示了实验的架构
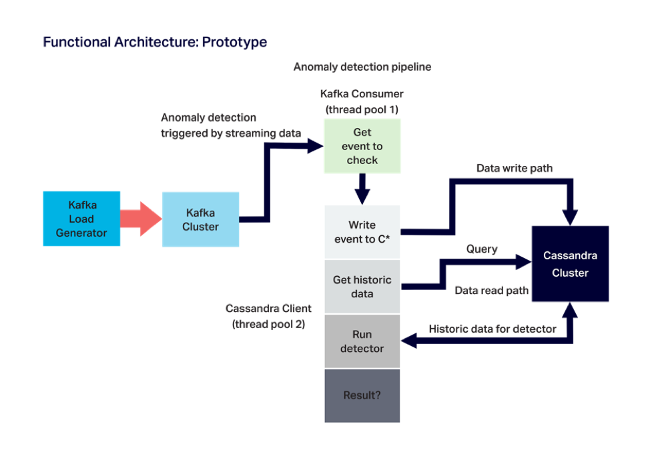
我们使用 Prometheus 的目标包括监控应用程序的更通用指标(例如吞吐量),以及 Kafka 负载生成器(Kafka 生产者)、Kafka 消费者和负责检测数据中任何异常的 Cassandra 客户端所提供的响应时间。 Prometheus 还监控系统的硬件指标,例如运行应用程序的每个 AWS EC2 实例的 CPU。 该项目还依赖 Prometheus 来监控特定于应用程序的指标,例如每个 Cassandra 读取返回的总行数,以及至关重要的是,它检测到的异常数量。 为简单起见,所有这些监控都是集中式的。
在实践中,这意味着形成一个具有生产者、消费者和检测器方法的测试管道,以及以下三个指标
- 每当每个管道阶段顺利执行时,一个名为 prometheusTest_requests_total 的计数器指标就会递增,而 stage 标签允许跟踪每个阶段的成功执行,而 total 标签跟踪总管道计数。
- 另一个计数器指标,名为 prometheusTest_anomalies_total,计算任何检测到的异常。
- 最后,一个名为 prometheusTest_duration_seconds 的仪表指标跟踪每个阶段的持续时间(再次使用 stage 标签和 total 标签)。
这些测量背后的代码使用 inc() 方法递增计数器指标,并使用 setToTime() 方法设置仪表指标的时间值。 这在以下带注释的示例代码中进行了演示
import java.io.IOException;
import io.prometheus.client.Counter;
import io.prometheus.client.Gauge;
import io.prometheus.client.exporter.HTTPServer;
import io.prometheus.client.hotspot.DefaultExports;
// https://github.com/prometheus/client_java
// Demo of how we plan to use Prometheus Java client to instrument Anomalia Machina.
// Note that the Anomalia Machina application will have Kafka Producer and Kafka consumer and rest of pipeline running in multiple separate processes/instances.
// So metrics from each will have different host/port combinations.
public class PrometheusBlog {
static String appName = "prometheusTest";
// counters can only increase in value (until process restart)
// Execution count. Use a single Counter for all stages of the pipeline, stages are distinguished by labels
static final Counter pipelineCounter = Counter.build()
.name(appName + "_requests_total").help("Count of executions of pipeline stages")
.labelNames("stage")
.register();
// in theory could also use pipelineCounter to count anomalies found using another label
// but less potential for confusion having another counter. Doesn't need a label
static final Counter anomalyCounter = Counter.build()
.name(appName + "_anomalies_total").help("Count of anomalies detected")
.register();
// A Gauge can go up and down, and is used to measure current value of some variable.
// pipelineGauge will measure duration in seconds of each stage using labels.
static final Gauge pipelineGauge = Gauge.build()
.name(appName + "_duration_seconds").help("Gauge of stage durations in seconds")
.labelNames("stage")
.register();
public static void main(String[] args) {
// Allow default JVM metrics to be exported
DefaultExports.initialize();
// Metrics are pulled by Prometheus, create an HTTP server as the endpoint
// Note if there are multiple processes running on the same server need to change port number.
// And add all IPs and port numbers to the Prometheus configuration file.
HTTPServer server = null;
try {
server = new HTTPServer(1234);
} catch (IOException e) {
e.printStackTrace();
}
// now run 1000 executions of the complete pipeline with random time delays and increasing rate
int max = 1000;
for (int i=0; i < max; i++)
{
// total time for complete pipeline, and increment anomalyCounter
pipelineGauge.labels("total").setToTime(() -> {
producer();
consumer();
if (detector())
anomalyCounter.inc();
});
// total pipeline count
pipelineCounter.labels("total").inc();
System.out.println("i=" + i);
// increase the rate of execution
try {
Thread.sleep(max-i);
} catch (InterruptedException e) {
e.printStackTrace();
}
}
server.stop();
}
// the 3 stages of the pipeline, for each we increase the stage counter and set the Gauge duration time
public static void producer() {
class Local {};
String name = Local.class.getEnclosingMethod().getName();
pipelineGauge.labels(name).setToTime(() -> {
try {
Thread.sleep(1 + (long)(Math.random()*20));
} catch (InterruptedException e) {
e.printStackTrace();
}
});
pipelineCounter.labels(name).inc();
}
public static void consumer() {
class Local {};
String name = Local.class.getEnclosingMethod().getName();
pipelineGauge.labels(name).setToTime(() -> {
try {
Thread.sleep(1 + (long)(Math.random()*10));
} catch (InterruptedException e) {
e.printStackTrace();
}
});
pipelineCounter.labels(name).inc();
}
// detector returns true if anomaly detected else false
public static boolean detector() {
class Local {};
String name = Local.class.getEnclosingMethod().getName();
pipelineGauge.labels(name).setToTime(() -> {
try {
Thread.sleep(1 + (long)(Math.random()*200));
} catch (InterruptedException e) {
e.printStackTrace();
}
});
pipelineCounter.labels(name).inc();
return (Math.random() > 0.95);
}
}
Prometheus 通过轮询(“抓取”)检测代码来收集指标(与其他一些通过推送方法接收指标的监控解决方案不同)。 上面的代码示例在端口 1234 上创建一个所需的 HTTP 服务器,以便 Prometheus 可以根据需要抓取指标。
以下示例代码解决了 Maven 依赖关系
<!-- The client -->
<dependency>
<groupId>io.prometheus</groupId>
<artifactId>simpleclient</artifactId>
<version>LATEST</version>
</dependency>
<!-- Hotspot JVM metrics-->
<dependency>
<groupId>io.prometheus</groupId>
<artifactId>simpleclient_hotspot</artifactId>
<version>LATEST</version>
</dependency>
<!-- Exposition HTTPServer-->
<dependency>
<groupId>io.prometheus</groupId>
<artifactId>simpleclient_httpserver</artifactId>
<version>LATEST</version>
</dependency>
<!-- Pushgateway exposition-->
<dependency>
<groupId>io.prometheus</groupId>
<artifactId>simpleclient_pushgateway</artifactId>
<version>LATEST</version>
</dependency>
下面的代码示例告诉 Prometheus 应该在哪里查找以抓取指标。 可以简单地将此代码添加到基本部署和测试的配置文件(默认值:Prometheus.yml)中。
global:
scrape_interval: 15s # By default, scrape targets every 15 seconds.
# scrape_configs has jobs and targets to scrape for each.
scrape_configs:
# job 1 is for testing prometheus instrumentation from multiple application processes.
# The job name is added as a label job=<job_name> to any timeseries scraped from this config.
- job_name: 'testprometheus'
# Override the global default and scrape targets from this job every 5 seconds.
scrape_interval: 5s
# this is where to put multiple targets, e.g. for Kafka load generators and detectors
static_configs:
- targets: ['localhost:1234', 'localhost:1235']
# job 2 provides operating system metrics (e.g. CPU, memory etc).
- job_name: 'node'
# Override the global default and scrape targets from this job every 5 seconds.
scrape_interval: 5s
static_configs:
- targets: ['localhost:9100']
请注意配置文件中名为“node”的作业,该作业使用端口 9100; 此作业提供节点指标,并且需要在运行应用程序的同一服务器上运行 Prometheus 节点 exporter。 应谨慎进行指标轮询:过于频繁会使应用程序过载,过于不频繁会导致滞后。 如果无法轮询应用程序指标,Prometheus 还提供了一个 推送网关。
查看 Prometheus 指标和结果
我们的实验最初使用表达式,后来使用Grafana 来可视化数据并克服 Prometheus 缺乏默认仪表板的问题。 使用 Prometheus 界面(或http://localhost:9090/metrics),按名称选择指标,然后将其输入表达式框以执行。 (请注意,在此阶段遇到错误消息是很常见的,因此如果您遇到一些问题,请不要气馁。)通过正确运行的表达式,结果将可用于在表格或图形中适当显示。
在计数器指标上使用 irate 或 rate 函数将生成有用的速率图
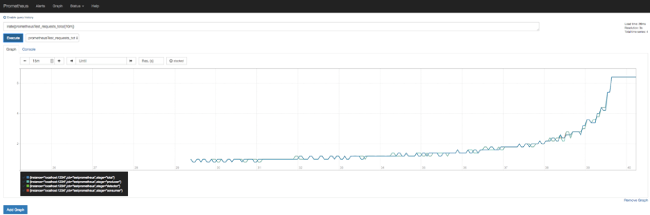
这是仪表指标的类似图表
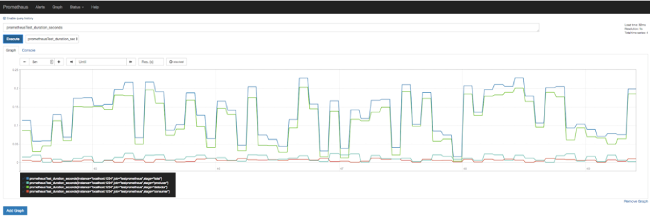
Grafana 提供了更强大的图形功能和内置的 Prometheus 支持,图形能够显示多个指标
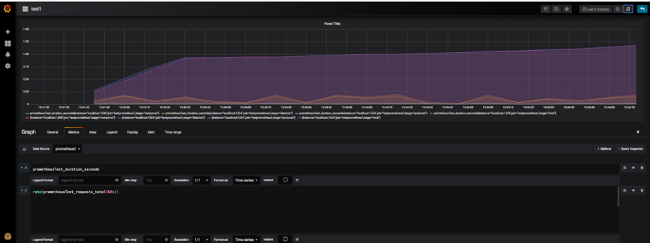
要启用 Grafana,请安装它,导航到 http://localhost:3000/,创建一个 Prometheus 数据源,并使用表达式添加一个 Prometheus 图。 此处要注意:空图通常指向时间范围问题,通常可以通过使用“最近 5 分钟”设置来解决。
创建此实验性应用程序为我们提供了绝佳的机会来构建我们对 Prometheus 功能的了解,并最终创建了一个高规模的实验性生产应用程序,该应用程序每天可以监控 190 亿个实时数据事件中的异常。 通过遵循本指南和我们的示例,希望更多的开发人员能够成功地将 Prometheus 投入实践。
4 条评论